Technological advances have ushered in a paradigm shift in how air quality data is measured and shared with the public. This change was due to the advent of low-cost sensors to measure gas and particle contaminants.
Air Pollutant Effects on Health
The International Agency for Research on Cancer (IARC) classifies ambient air pollution in group 1 with the definitive definition of carcinogen (IARC, 2013). The World Health Organization estimates that about 7 million people die yearly from air pollution worldwide. Furthermore, the number of premature deaths from air pollution is expected to double by 2050, and the burden of diseases caused by air pollution will increase steadily (EEA, 2023). Therefore, an air monitoring system is crucial to understanding the characteristics of pollutants in urban environments, taking action against problems associated with poor air quality, and assessing the effectiveness of initiatives designed to combat it.
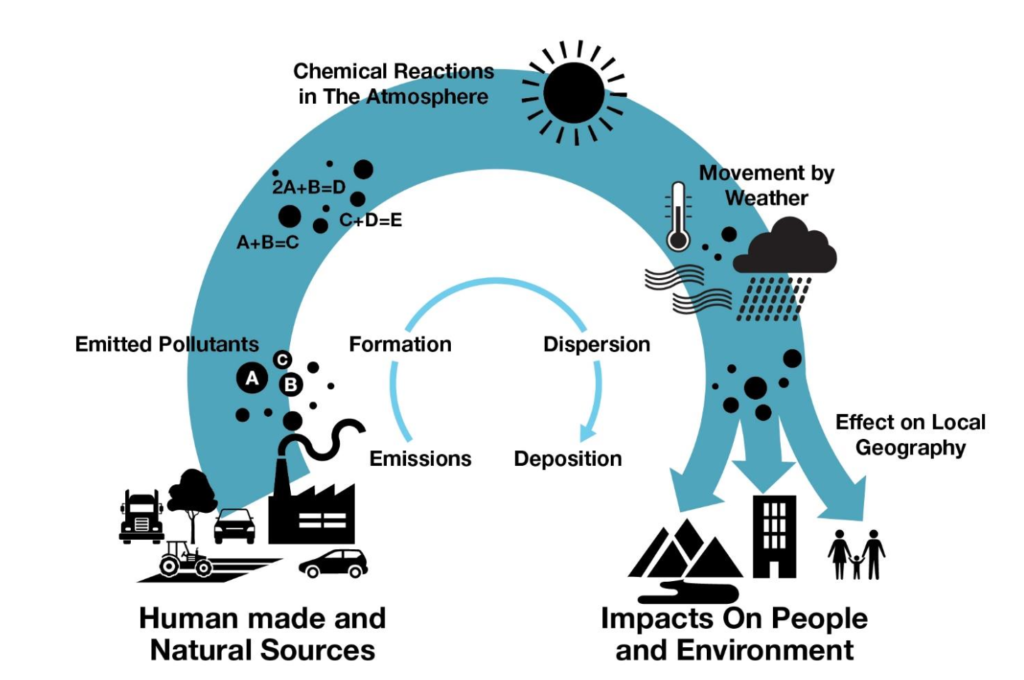
Air Pollution Monitoring
Traditionally, air pollutant concentrations are monitored using professional air quality monitoring stations that meet strict accuracy criteria. Such stations are susceptible but, at the same time, costly. Due to high distribution and operating costs, professional stations are installed infrequently, and in most metropolitan areas, there is only a single measuring station. While in line with official recommendations, more density is needed, as even a single city block can witness significant differences in pollutant concentrations. For example, congested traffic corridors like intersections or bus stops tend to have significantly higher pollution than surrounding areas (Concas, et al., 2021).
To accurately assess the health and environmental risks of pollutants, it is also necessary to understand the chemical composition of contaminants, which varies depending on the season and industry characteristics, and traffic in the region. For these reasons, accurate air pollution monitoring in metropolitan areas will require deploying hundreds or even thousands of air quality monitoring stations (Concas et al., 2021). In contrast, the WHO recommends the establishment of one air quality monitoring station per square kilometer, while the EU clean air directive recommends one station per approximately 200,000 people (EEA, 2008).
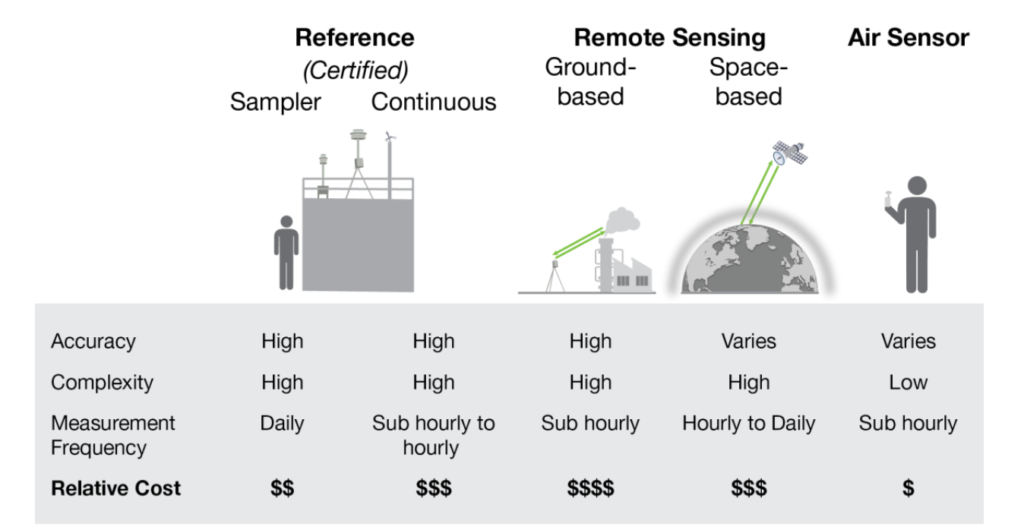
What Does Indicative Monitoring Mean?
Indicative monitoring is a category defined by EU Directive 2008/50/EC on ambient air quality monitoring. Applies to a measurement aligned with a data quality goal lower than the reference or close reference. Data generated via indicative monitoring can provide information on the spatial distribution of air pollutants and is instrumental in communicating current air quality to the public.
Low-cost sensors have emerged to provide accurate or near real-time contaminant information at increasing spatial resolutions with the potential to complement and expand the capabilities of existing ambient air monitoring networks and provide meaningful measurements at a local scale. In addition, the compact size of the low-cost sensors makes it possible to place them intensively as part of the urban infrastructure (Feenstra et al., 2019).
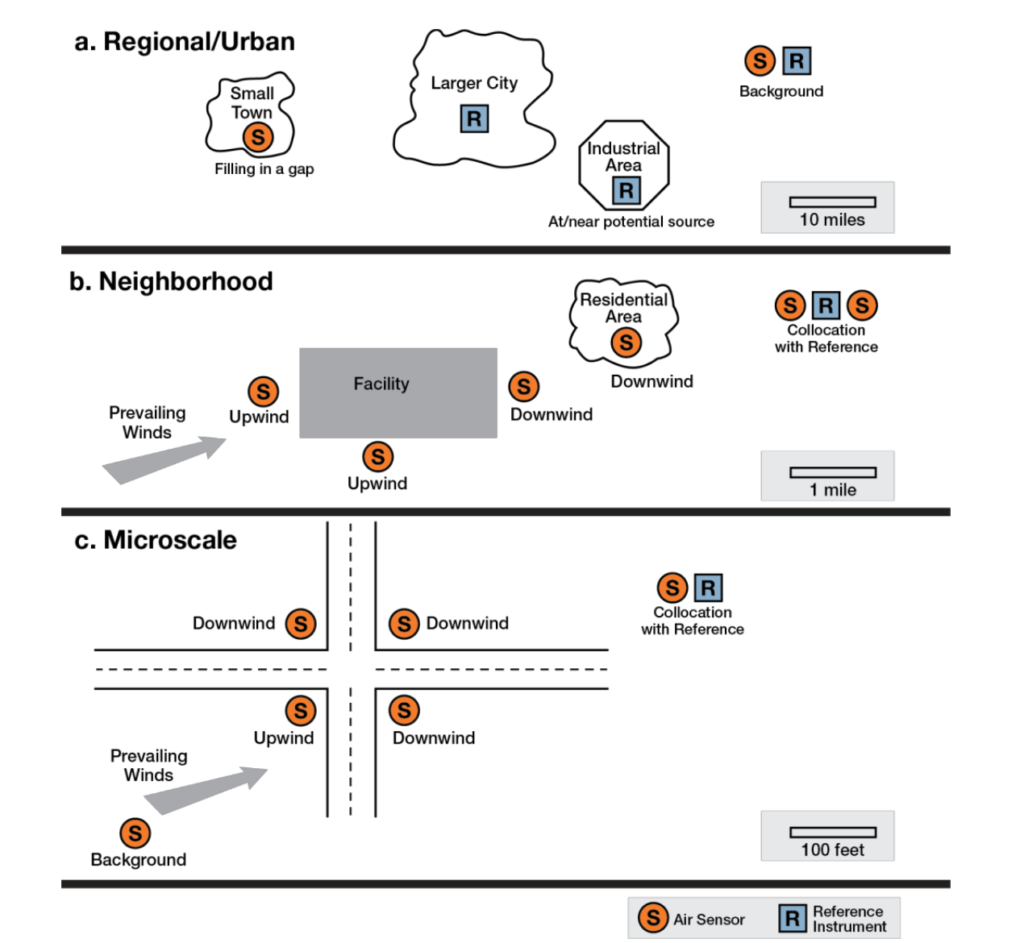
How Are The Performance Criteria of Air Sensors Defined?
The main disadvantage of low-cost air quality sensors is that their accuracy tends to be poor compared to reference tracking stations. The sensors’ accuracy, lifespan, and reliability vary depending on the underlying measurement technology, the quality of the different air sensor components, environmental conditions, and operating methods. Systematic and comprehensive field and laboratory studies comparing low-cost sensors to regulatory-grade instrumentation are required to characterize sensor performance (Feenstra, et al., 2019).
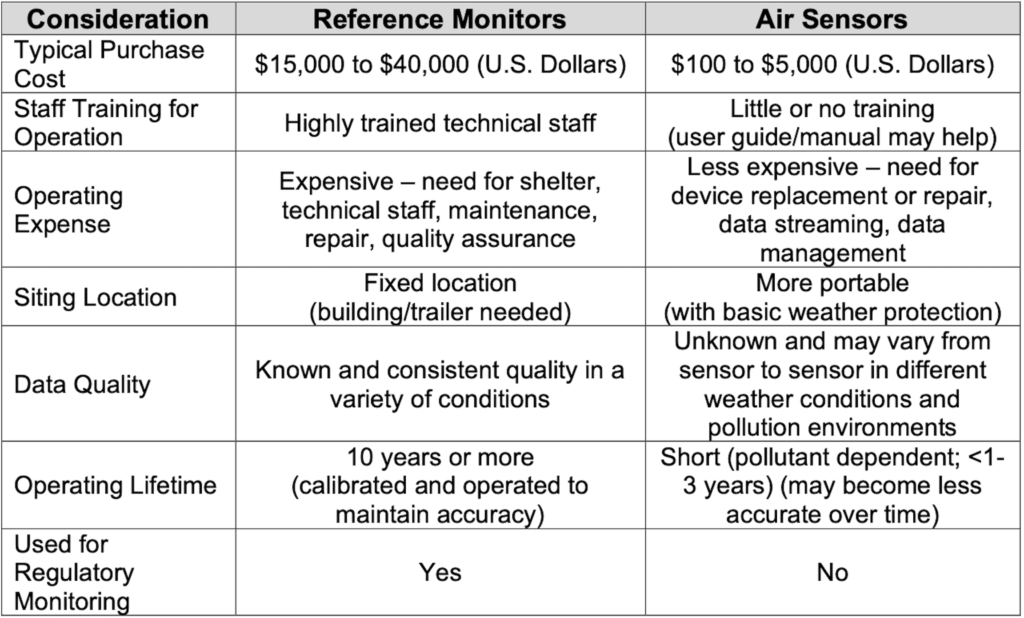
For performance criteria used to evaluate air quality modeling applications, they include root mean square error (RMSE), bias, standard deviation (SD), and correlation coefficient (R2). Average Square Error gives you an absolute number of how different your predicted results are from the actual number (Karagulian et al., 2019). RMSE is the root of the average square error of the measurement error between the sensor and the reference data; it is a frequently applied accuracy statistic and is very dependent on peaks/flows. The smaller the RMSE, the better (Hoffman et al., 2022).
An R2 value indicates how closely a sensor’s data is related to Federal Reference Methods (FRM)/Federal Equivalent Methods (FEM) concentration measurements. In other words, R2 is a statistical term that represents how well a sensor performs compared to reference tracking technologies. For example, a value of R2=1 means that the sensor is perfectly correlated with the reference monitor, and R2=0 means that there is no correlation with the reference monitor (Dybwad, 2022). The determination coefficient of R2 > 0.75 is the best weather sensor that can be used to monitor air quality pollutants (Karagulian et al., 2019).
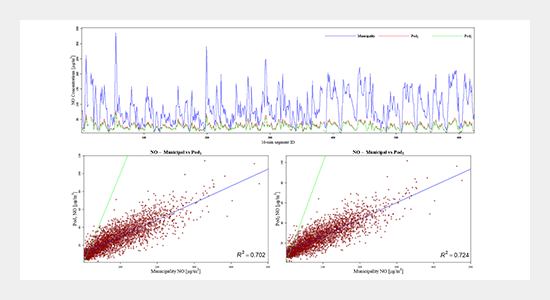
Periodic calibration alone is insufficient for the accuracy of low-cost sensors because the sensors are vulnerable to changes in atmospheric conditions such as temperature, humidity, and wind direction, as examples of cross-sensitivities between different contaminants and factors that affect sensor performance. Despite the fact that sensors of the same type often perform similarly, it is recommended that this cannot be guaranteed. Therefore a primitive sensor-to-sensor comparison is always carried out in the intended environment. To maintain high data quality, all long-term air quality measurements must be regularly checked and maintained (Watne et al., 2021).
There is currently no standard protocol in the world for testing air sensors against reference measurement. Recognizing the need for a coherent approach to assessing air sensor performance, the U.S. EPA published reports in 2021 that make recommendations on how to evaluate air sensors that measure benchmark pollutants. The Air Quality Directive 2008/50/EC is being updated this year. It is expected that this Directive will address the use and evaluation of sensors.
References
Concas, F., Mineraud, J., Lagerspetz, E., Varjonen, S., Liu, X., Puolamaki, K., . . . Tarkoma, S. (2021). Low-Cost Outdoor Air Quality Monitoring and Sensor Calibration: A Survey and Critical Analysis. ACM Transactions on Sensor Networks (TOSN), 17(2), 1-44.
Dybwad, A. (2022). What are R2 values in Air Quality? Purple Air.
EEA. (2008). Directive 2008/50/EC, Air Quality.
EEA. (2023). Premature Deaths Due to Air Pollution Continue to Fall in The EU, More Efforts Needed to Deliver A Toxic-Free Environment.
EPA. (2021). Performance Testing Protocols, Metrics, and Target Values for Fine Particulate Matter Air Sensors Use in Ambient, Outdoor, Fixed Site, Non-Regulatory Supplemental and Informational Monitoring Applications. EPA/600/R-20/280.
EPA. (2022). The Enhanced Air Sensor Guidebook.
Feenstra, B., Papapostolou, V., Hasheminassab, S., Zhang, H., Boghossian, B. D., Cocker, D., & Polidori, A. (2019). Performance Evaluation of Twelve Low-Cost PM2.5 Sensors at An Ambient Air Monitoring Site. Atmospheric Environment, 216, 116946.
Hofman, J., Peters, J., Stroobants, C., Elst, E., Baeyens, B., Laer, J. V., . . . Poppel, M. V. (2022). Air Quality Sensor Networks for Evidence-Based Policy Making: Best Practices for Actionable Insights. Atmosphere, 13(6), 944.
IARC. (2013). Outdoor Air Pollution A Leading Environmental Cause of Cancer Deaths.
Karagulian , F., Barbiere , M., Kotsev, A., Spinelle , L., Gerboles , M., Lagler, F., . . . Borowiak, A. (2021). Review of the Performance of Low-Cost Sensors for Air Quality Monitoring. Atmosphere, 10(9), 506.
Watne, Å., Linden, J., Willhelmsson, J., Fridén, H., Gustafsson, M., & Castell, N. (2021). Tackling Data Quality When Using Low-Cost Air Quality Sensors in Citizen Science Projects. Frontiers in Environmental Science, 9, 733634.